Implementation of an enhanced target localization and identification algorithm on a magnetic WSN
This work presents the development and implementation of an enhanced magnetic sensing algorithm for target localization and identification within sparse wireless sensor networks (WSNs). Designed to operate with energy-efficient hardware in real-world scenarios, the proposed system employs Honeywell HMC1002 2-axis magnetometers integrated into MICAz sensor nodes. The core innovation lies in leveraging Orthogonal Matching Pursuit (OMP) to achieve fine-grained localization (within 10 cm resolution) and target identification based on magnetic field signatures—all from a single magnetic sensor node.
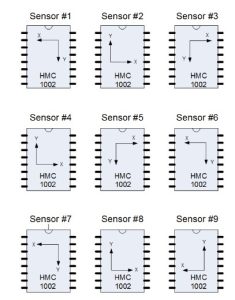
The system is specifically designed for sparse deployments, where sensing regions of magnetic sensors do not overlap. This architecture reduces network cost and energy consumption while posing challenges in tracking accuracy, especially in blind zones. By constructing a dictionary of magnetic field measurements across different positions and target types, the OMP algorithm can infer both the target’s location and identity, even when only partial magnetic data is available.
Through extensive experimentation, the study investigates practical constraints such as power supply variation, ambient temperature changes, sensor sensitivity variation, and magnetic noise—factors that significantly affect the accuracy of magnetic measurements. Adaptive counter-based filtering and calibration strategies are introduced to mitigate these challenges, ensuring robustness in dynamic environments.
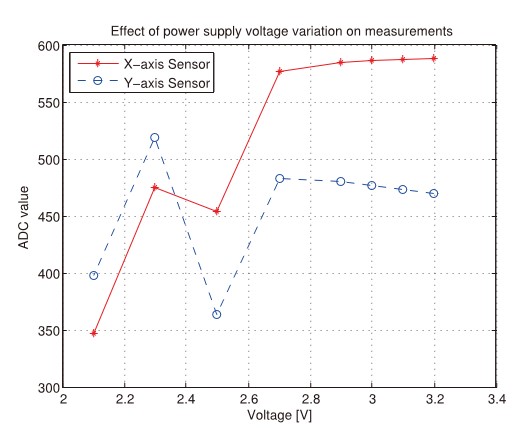
Experimental validation is conducted both in laboratory settings—with iron targets of varying sizes and configurations—and in an outdoor testbed for real-time vehicle localization in a parking lot. The system achieves a 72% accuracy in fine-grained 28-cell resolution localization and 100% accuracy at quadrant-level resolution. Furthermore, identification accuracy improves significantly with prior knowledge of entry location, reaching perfect classification when the target’s entry point is known.
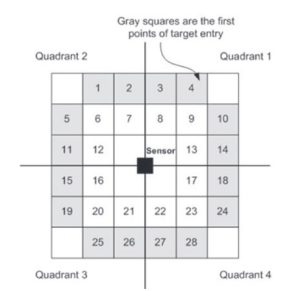
By enabling localization and classification from a single sensor reading, this study lays the groundwork for more scalable and energy-aware WSN designs. The algorithm’s lightweight computational requirements and compatibility with real-time systems make it suitable for applications in smart traffic systems, parking lot management, and security monitoring.
This work contributes to the growing body of energy-efficient, intelligent sensing solutions in the Internet of Things (IoT) ecosystem and highlights the practical feasibility of using sparse sensor deployments for accurate magnetic-based tracking and classification.
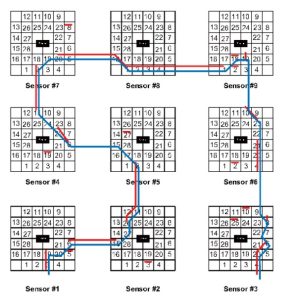
Link 1:
For more information, please click here!
Link 2: