User comfort and energy efficiency in HVAC systems by Q-learning
This research introduces an intelligent control strategy for Heating, Ventilation, and Air Conditioning (HVAC) systems based on Q-learning—a model-free reinforcement learning technique—to simultaneously optimize user comfort and energy efficiency in indoor environments. The core objective is to regulate indoor air quality (IAQ), specifically carbon dioxide (CO₂) concentration, by dynamically adjusting the ventilation rate of the HVAC system in response to occupant density and environmental changes.
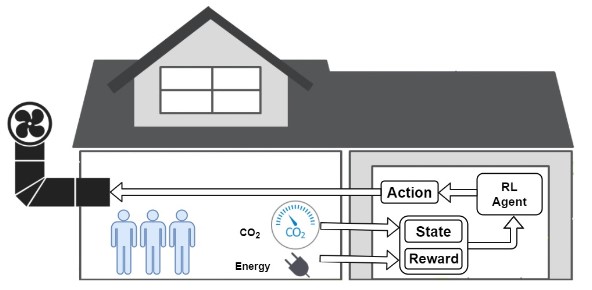
Unlike traditional On-Off and fixed setpoint control methods, the proposed Q-learning framework enables the HVAC agent to learn optimal actions over time by interacting with the environment and receiving feedback through a custom-designed reward function. This reward balances two competing goals: minimizing energy consumption and keeping CO₂ levels close to a comfort-driven target (800 ppm). The agent’s policy is shaped through iterative updates to a Q-matrix, representing the learned knowledge of state-action values, without requiring a predefined environmental model.
The methodology discretizes CO₂ concentration levels into states and HVAC ventilation rates into actions. Through exploration and exploitation, the system continuously adapts its control policy under varying occupancy levels and CO₂ generation rates. Simulation results demonstrate that the Q-learning method effectively balances energy use and indoor air quality, achieving better performance than both On-Off and Setpoint control strategies. Specifically, it reduces energy consumption during unoccupied periods while preserving air quality during occupancy.
A case study involving a 450 m³ room with a dynamic occupancy schedule validates the approach. Compared to baseline controllers, the Q-learning controller maintains CO₂ concentrations near the desired level with significantly lower energy usage, especially when a balance parameter (β) is tuned to prioritize comfort or energy as needed. The system shows robust convergence behavior for various learning rates and discount factors, ensuring adaptability to different building dynamics.
In conclusion, the proposed Q-learning HVAC controller presents a scalable, adaptive, and energy-conscious solution for next-generation smart buildings. It enhances occupant comfort while contributing to sustainability goals by reducing unnecessary HVAC energy expenditure. Future extensions may include integration with temperature and humidity control, deep reinforcement learning models, and deployment in real-world testbed.